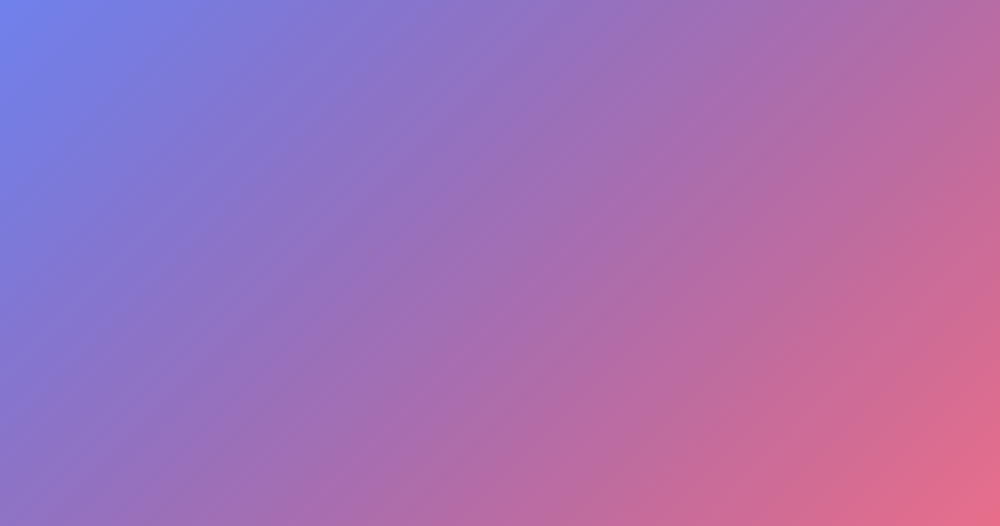
3
febreroIt was Trained For Logical Inference
In case you haven’t been paying consideration, one thing monstrous has emerged in the AI panorama : DeepSeek. Surely DeepSeek did this. For comparison, Meta AI's Llama 3.1 405B (smaller than DeepSeek v3's 685B parameters) trained on 11x that - 30,840,000 GPU hours, also on 15 trillion tokens. The Financial Times reported that it was cheaper than its peers with a worth of two RMB for each million output tokens. At solely $5.5 million to train, it’s a fraction of the cost of models from OpenAI, Google, or Anthropic which are often within the a whole lot of millions. The collection includes four models, 2 base fashions (DeepSeek-V2, DeepSeek-V2-Lite) and a pair of chatbots (-Chat). The corporate additionally launched some "DeepSeek-R1-Distill" fashions, which aren't initialized on V3-Base, but instead are initialized from different pretrained open-weight fashions, including LLaMA and Qwen, then effective-tuned on artificial data generated by R1. Each skilled mannequin was trained to generate simply artificial reasoning data in a single particular area (math, programming, logic). The "expert fashions" have been skilled by starting with an unspecified base model, then SFT on both information, and synthetic knowledge generated by an inside DeepSeek-R1 model. DeepSeek's competitive efficiency at comparatively minimal value has been acknowledged as potentially difficult the worldwide dominance of American AI fashions.
Various publications and information media, such as the Hill and The Guardian, described the release of its chatbot as a "Sputnik moment" for American AI. Its chatbot reportedly solutions questions, solves logic problems, and writes computer packages on par with different chatbots on the market, in accordance with benchmark tests utilized by American AI corporations. American Silicon Valley enterprise capitalist Marc Andreessen likewise described R1 as "AI's Sputnik second". The success here is that they’re related amongst American technology corporations spending what's approaching or surpassing $10B per year on AI fashions. free deepseek claimed that it exceeded performance of OpenAI o1 on benchmarks corresponding to American Invitational Mathematics Examination (AIME) and MATH. DeepSeek-Prover, the mannequin skilled by means of this method, achieves state-of-the-artwork performance on theorem proving benchmarks. First, they fine-tuned the DeepSeekMath-Base 7B model on a small dataset of formal math issues and their Lean four definitions to acquire the preliminary model of DeepSeek-Prover, their LLM for proving theorems. Turning small fashions into reasoning models: "To equip extra environment friendly smaller fashions with reasoning capabilities like DeepSeek-R1, we straight tremendous-tuned open-supply models like Qwen, and Llama using the 800k samples curated with DeepSeek-R1," DeepSeek write.
When evaluating model outputs on Hugging Face with these on platforms oriented in direction of the Chinese audience, models subject to much less stringent censorship provided extra substantive solutions to politically nuanced inquiries. The built-in censorship mechanisms and restrictions can only be eliminated to a restricted extent in the open-source version of the R1 model. It's also possible to make use of vLLM for prime-throughput inference. I will consider adding 32g as nicely if there's interest, and as soon as I've carried out perplexity and evaluation comparisons, but right now 32g fashions are nonetheless not totally examined with AutoAWQ and vLLM. They lowered communication by rearranging (each 10 minutes) the exact machine every expert was on in order to keep away from sure machines being queried extra usually than the others, adding auxiliary load-balancing losses to the training loss perform, and different load-balancing strategies. They found this to help with skilled balancing. Expert models have been used, as an alternative of R1 itself, for the reason that output from R1 itself suffered "overthinking, poor formatting, and extreme length". For Budget Constraints: If you're limited by price range, deal with free deepseek GGML/GGUF fashions that match inside the sytem RAM. RAM needed to load the mannequin initially. 4. The mannequin will begin downloading.
Today, we are going to find out if they'll play the sport in addition to us, as nicely. The particular questions and check circumstances can be released soon. For example, the model refuses to answer questions concerning the 1989 Tiananmen Square massacre, persecution of Uyghurs, comparisons between Xi Jinping and Winnie the Pooh, and human rights in China. This knowledge includes helpful and impartial human instructions, structured by the Alpaca Instruction format. Finally, the replace rule is the parameter replace from PPO that maximizes the reward metrics in the current batch of information (PPO is on-coverage, which means the parameters are solely updated with the current batch of immediate-generation pairs). The reward model was repeatedly up to date throughout training to avoid reward hacking. "Behaviors that emerge whereas training brokers in simulation: searching for the ball, scrambling, and blocking a shot… What the brokers are fabricated from: These days, more than half of the stuff I write about in Import AI entails a Transformer architecture mannequin (developed 2017). Not here! These brokers use residual networks which feed into an LSTM (for memory) after which have some fully linked layers and an actor loss and MLE loss.
Reseñas